Data breaches within U.S. government agencies resulted in an estimated $26 billion in losses over the past eight years, with incidents such as those at the U.S. Postal Service and the Office of Personnel Management (OPM) exposing millions of sensitive records. While artificial intelligence (AI) is being adopted across federal operations, a previous study from the Government Accountability Office (GAO) highlighted uneven implementation—NASA leads with 390 AI use cases, while agencies like the OPM and EPA report only 1 to 4 cases, underscoring the urgent need for AI-driven solutions to enhance data security and operational efficiency.
A promising advancement is Retrieval-Augmented Generation (RAG), a technology that integrates real-time data with AI-generated responses, improving accuracy and relevance. For federal agencies, particularly in critical sectors like homeland security or healthcare, RAG provided as a service offers the potential to bridge the gap in AI adoption, providing more secure and compliant solutions to ensure data integrity and compliance with regulatory frameworks.
Limitations of Current Large Language Models (LLMs)
Large Language Models (LLMs), such as GPT-3 and GPT-4, excel at generating human-like text based on patterns learned from extensive datasets. However, they face significant limitations in government settings, particularly in handling sensitive, real-time data. These models are trained on static data, which means their knowledge is often outdated, leading to inaccuracies when responding to queries that require current information. Furthermore, LLMs are prone to generating “hallucinations“—responses that seem plausible but are incorrect or nonsensical. In federal agencies, such hallucinations can result in misinformation, impacting decision-making processes and undermining trust in mission-critical AI systems.
Additionally, some LLMs lack transparency and traceability in their reasoning processes, making it difficult to understand how they arrive at certain conclusions. This “black box” nature poses risks for government agencies that must ensure accountability, data integrity, and compliance with regulatory standards. LLMs are unsuitable for critical applications like healthcare, security, and policy-making without appropriate safeguards, such as data validation mechanisms and contextual grounding. These limitations highlight the need for advanced solutions like RAG to mitigate risks and improve accuracy in government AI deployments.
Understanding Retrieval-Augmented Generation (RAG)
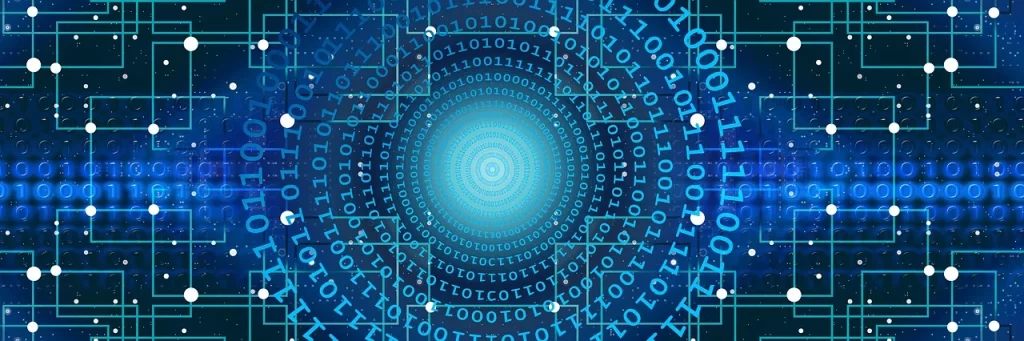
RAG enhances generative AI by connecting it to external data sources, providing real-time context to improve the accuracy of responses. By integrating structured data through taxonomies, ontologies, and knowledge graphs, RAG helps AI models generate more accurate, contextually relevant responses and reduces hallucinations. Key components of RAG include:
- Contextual Data Enrichment: Leverages organization-specific taxonomies to provide AI with deeper contextual understanding.
- Knowledge Graphs: Organizes and uncovers connections within data, ensuring that AI responses are based on precise information.
- Prompt Enhancement: Frames user queries using knowledge graphs, ensuring precise and context-aware answers.
- Response Validation: AI outputs are validated against knowledge models to ensure accuracy and reliability.
Challenges of Implementing LLM in Government Settings
Implementing LLM in government settings presents unique challenges due to the sensitivity of classified data and the need for strict access controls. Federal agencies require paragraph-level data classification rather than document-level to ensure only authorized information is accessed. This significantly complicates AI deployment. Moreover, robust security features like advanced access control, auditing, and monitoring are essential to meet regulatory compliance standards. Commercial RAG solutions often need more fine-grained control and security features for government applications. This gap highlights the need for specialized solutions tailored to meet the rigorous data protection and compliance requirements of federal agencies.
The Importance of Tailored RAG Solutions
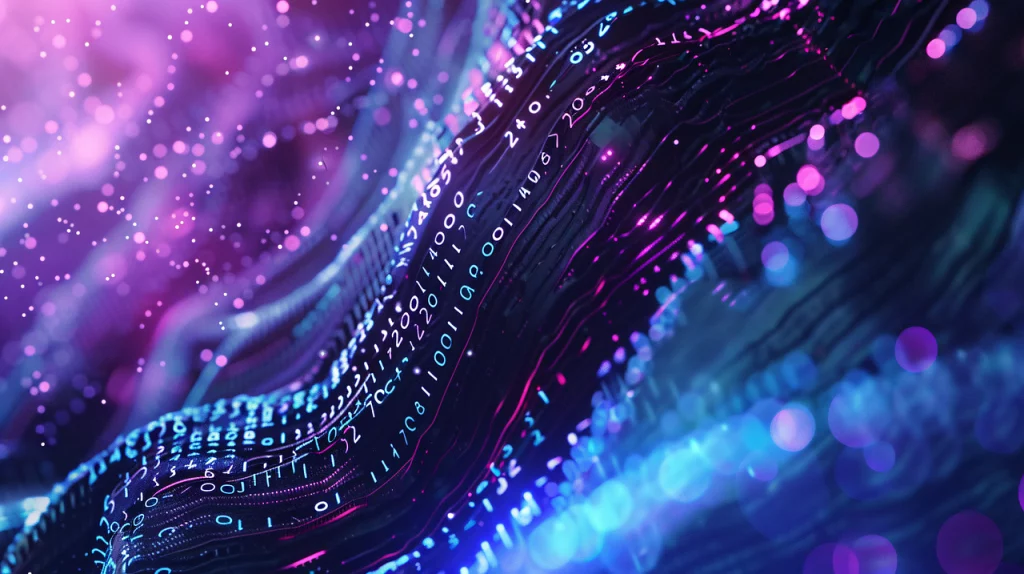
Developing tailored RAG solutions is crucial to bridge the gap between commercial AI solutions and government-specific needs. Key features include:
- Enhanced Data Classification and Stringent Access Control: Fine-grained, paragraph-level control ensures that only authorized data segments are accessed, combined with comprehensive oversight aligned with federal security policies.
- Regulatory Alignment and Compliance: Built-in frameworks ensure adherence to government-specific regulations, with automated compliance checks to assess and reduce non-compliance risks.
- Mitigating Security Risks: Access Control Lists (ACLs) restrict access to classified data, ensuring that only authorized personnel can view or modify sensitive information, preventing unauthorized data access and breaches.
- Seamless Integration: Solutions must integrate with common platforms like Microsoft Teams and Slack, making it easy for federal agencies to securely access and share data across their existing communication systems while ensuring compliance with government data protection standards.
Use Case: Federal Healthcare
The Center for Medicare and Medicaid Services (CMS) is working to modernize healthcare delivery by integrating advanced health IT solutions. RAG can play a key role by augmenting clinical decision support systems. For example, GPT-4 turbo combined with RAG has been used to manage bipolar depression by integrating clinical guidelines and evidence-based data in real time, improving diagnosis and treatment recommendations. This application shows how RAG enhances both the specificity and accuracy of AI responses, reducing errors in critical healthcare decisions. This serves as a model for broader RAG adoption across other agencies, allowing for accurate data retrieval and improved decision-making in complex and regulated environments.
Conclusion
Retrieval-Augmented Generation provides federal agencies with a secure, compliant, and efficient AI solution for enhancing decision-making and data management. By integrating real-time data and automating processes, RAG addresses the unique challenges government entities face in protecting sensitive data and maintaining regulatory compliance.