Assessing AI readiness is fundamental for government agencies to ensure that the deployment and integration of artificial intelligence align with their strategic goals and enhance operational efficiencies. This evaluation is key to mitigating risks associated with AI implementation failures and avoiding investments that could derail agency objectives. A comprehensive assessment examines an agency’s capabilities across strategy, data, processes, and governance—essential for preparing the groundwork for successful AI deployment.
In alignment with the Executive Order on the Safe, Secure, and Trustworthy Development and Use of Artificial Intelligence, agencies must adhere to rigorous evaluation and governance frameworks. This adherence ensures that AI systems are ethically developed, operationally secure, and compliant with federal laws, thereby supporting the broader goals of enhancing national security, economic prosperity, and public welfare through responsible AI use.
Critical components
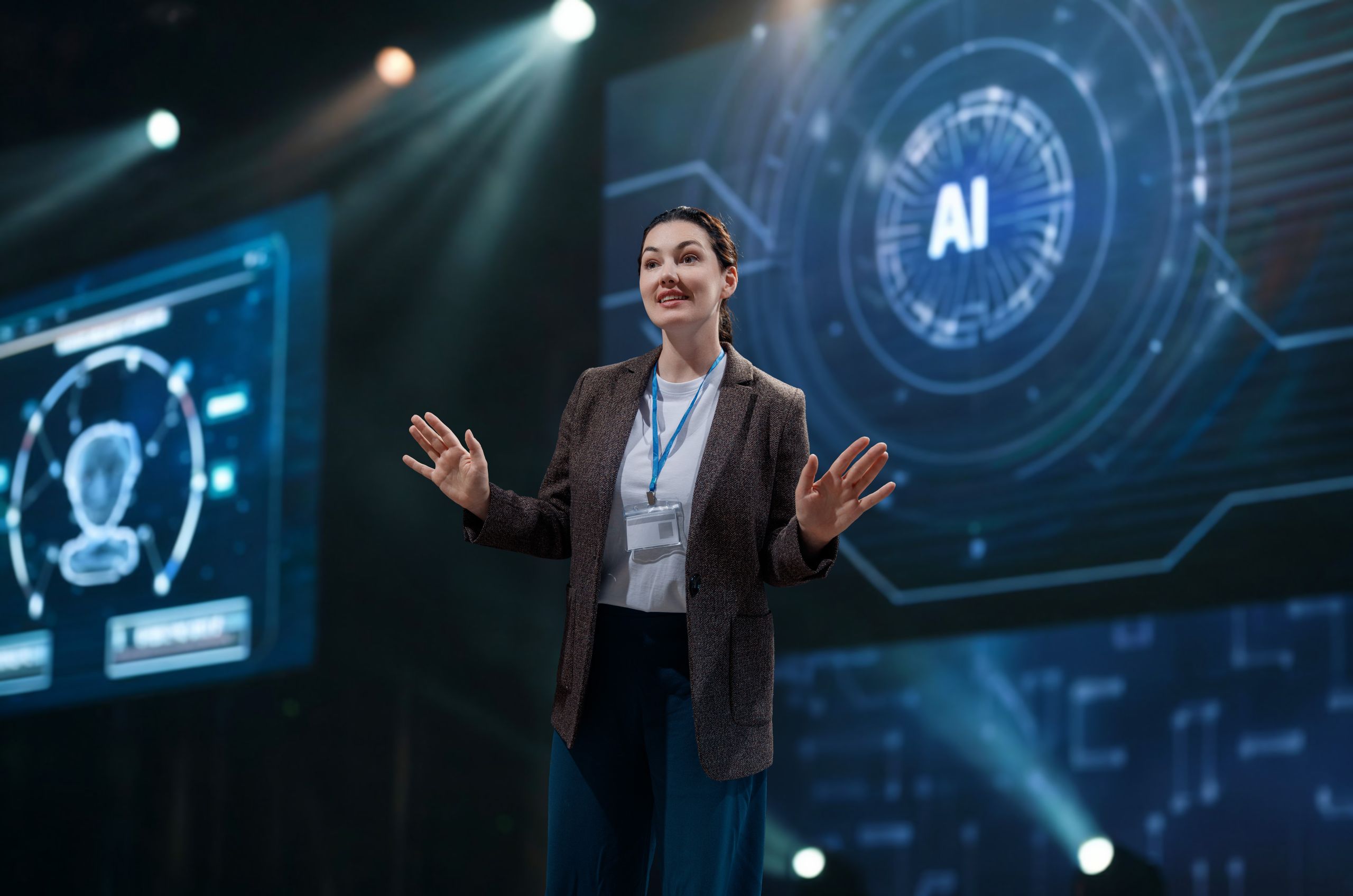
An agency should consider six critical components of AI readiness: Strategy, People, Processes, Data, Technology & Platforms, and Ethics. Each component is vital for the successful implementation of AI, helping agencies develop a nuanced understanding of their readiness and align all aspects from human capital to ethical standards with both their immediate needs and long-term strategic goals:
- Strategy: Ensures AI initiatives align with the agency’s long-term goals and operational tactics. It involves setting clear objectives for AI deployments and establishing measurable outcomes.
- People: This component emphasizes the workforce’s readiness to adopt and interact with AI technologies, including the importance of training programs to enhance AI literacy and the recruitment or development of specialized AI talent. The AI Talent Surge Progress Report illustrates that federal agencies are already effectively implementing these strategies by actively recruiting and developing AI professionals – highlighting the critical role of preparedness in the successful integration of AI across government operations.
- Processes: Involves the adaptation of existing workflows to accommodate AI technologies. This includes the redesign of business processes for improved efficiency and effectiveness through automation.
- Data: Critical for training and running AI models. Emphasizes the importance of data quality, accessibility, and the infrastructure required to handle large datasets securely and efficiently.
- Technology & Platforms: Pertains to the hardware and software that underpin AI systems. This includes the integration with existing IT infrastructure and the evaluation of new technologies that support AI capabilities.
- Ethics: Addresses the moral implications of AI deployment, focusing on developing frameworks that ensure transparency, fairness, and accountability. Includes guidelines to mitigate biases and protect privacy.
Each component must be robustly developed and closely interlinked to ensure AI initiatives are not only implementable but also sustainable and scalable within the agency’s ecosystem. The model advocates for a methodical approach to accessing AI readiness where each aspect of government AI integration is carefully planned and aligned with strategic organizational goals.
1. Strategy
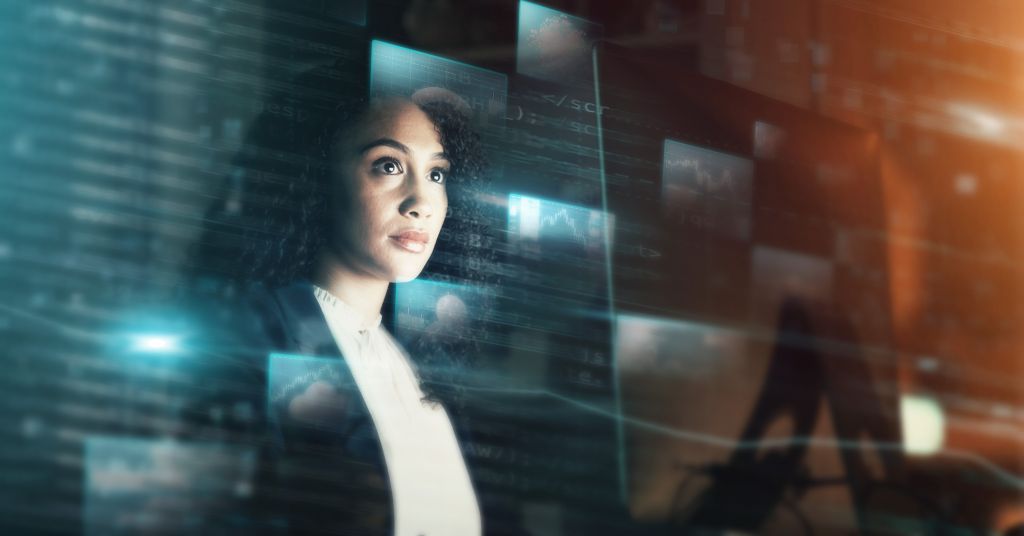
AI adoption across government agencies has rapidly expanded, with nearly half of federal agencies experimenting with AI by 2020 and about 1,200 distinct AI use cases identified by 2023. Despite this widespread use in functions like law enforcement, public benefit administration, and housing, the flaws in many AI use cases raise questions about the need for a well-developed AI strategy and roadmap: how can tailored strategies align with agency goals to ensure effective and responsible AI readiness?
Aligning AI With Agency Goals
The first step in executing an AI use case is to ensure that AI initiatives are directly aligned with the agency’s statutory mission and policy mandates. This involves translating the agency’s strategic objectives into AI capabilities, such as enhancing citizen service delivery through automated support systems, improving regulatory compliance with AI-driven analytics, or optimizing resource distribution by utilizing predictive algorithms.
For AI to add value, it must be embedded in a way that can support critical agency functions. Government agencies need to strategically allocate resources by conducting readiness assessments across all sectors, focusing on areas like cybersecurity, public safety, healthcare, and infrastructure. This ensures that investment in AI delivers measurable operational gains and minimizes risks by being aligned with high-priority goals. For instance, AI could be deployed to enhance fraud detection in social welfare programs, where resource allocation can directly reduce financial mismanagement.
Developing a Future-Focused Strategy
Agencies must adopt a proactive approach to include emerging AI trends in their strategic plans. This includes:
- AI-driven chatbots for citizen inquiries.
- Automated document processing for public records.
- Advanced machine learning models to predict public health crises.
Staying updated on developments in AI-driven automation and its applicability to public administration ensures that agencies remain capable of adapting to and integrating new technologies to enhance service delivery.
Strategic Integration with Existing Technology Frameworks
Integrating AI into outdated or complex legacy IT systems remains one of the most significant challenges for government agencies. In 2023, the GAO reported that several agencies were still struggling to modernize critical legacy systems—many of which continue to operate on outdated technologies, such as COBOL, and collectively cost about $337 million annually to maintain.
To effectively leverage AI, agencies must prioritize modernization efforts so that new AI capabilities can be integrated into updated systems, ensuring seamless interoperability. This modernization may involve leveraging Application Programming Interfaces (APIs) to connect AI tools to legacy databases or integrating AI models in stages to ensure compatibility and minimize risks.
2. People
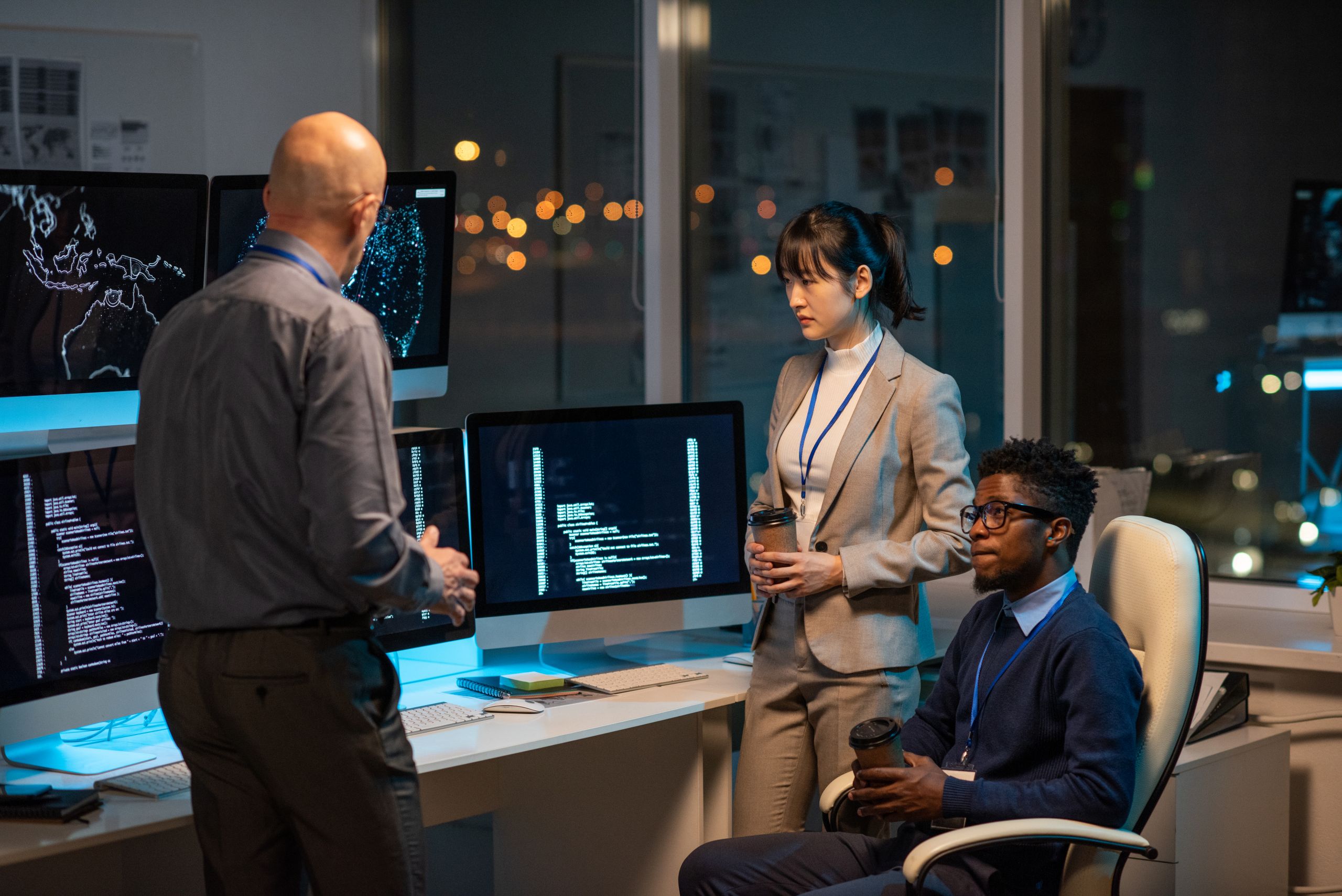
Workforce Readiness and AI Talent Development
The readiness of the workforce to adopt and interact with AI technologies is critical. This involves implementing training programs to enhance AI literacy and recruiting or developing specialized AI talent. Bridging the skills gap through targeted strategies helps government agencies develop their workforce and ensure employees can effectively leverage AI, fostering both foundational and specialized skills to support responsible AI adoption.
Currently, federal agencies are responding to a critical demand for specialized skills through focused upskilling initiatives, aligned with President Biden’s October 2023 executive order on artificial intelligence. This directive mandates the development of ethical AI practices and the establishment of dedicated AI offices, aiming to equip the federal workforce for technological advancements and place them at the forefront of responsible AI implementation.
This strategic focus on workforce readiness is essential for evaluating AI preparedness within government agencies. By concentrating on building the necessary skills, agencies can ensure their workforce is prepared to effectively deploy, manage, and sustain AI capabilities, supporting successful, ethical AI integration across federal operations.
Cross-Functional Teams and Organizational Buy-in
Successful AI integration also relies on fostering organizational buy-in. It is crucial to align AI initiatives with the agency’s mission, goals, and strategic objectives to gain support from stakeholders. Establishing clear value propositions of AI and demonstrating tangible benefits from pilot projects help build internal advocacy. Continuous involvement of cross-functional teams—comprising IT, data management, and domain experts—also ensures that AI initiatives are seen as valuable contributors to the agency’s overall goals.
3. Processes
Processes need to be adapted to accommodate AI technologies, including redesigning business processes for improved efficiency and effectiveness through automation. This might involve integrating AI tools into existing workflows or adjusting operational processes to maximize AI’s impact.
Pilot AI projects must demonstrate their ability to effectively integrate into larger operational workflows while handling increased data input and broader deployment contexts. Government agencies need to assess how their AI initiatives will perform under increased demand, ensuring that the model’s accuracy and speed are maintained during scale-up.
The scalability of AI systems often hinges on the robustness of supporting data pipelines and the extent to which current infrastructure can accommodate AI. Addressing these challenges requires proactive solutions, such as upgrading data architecture and implementing scalable cloud-based systems to manage increased computational load.
4. Data
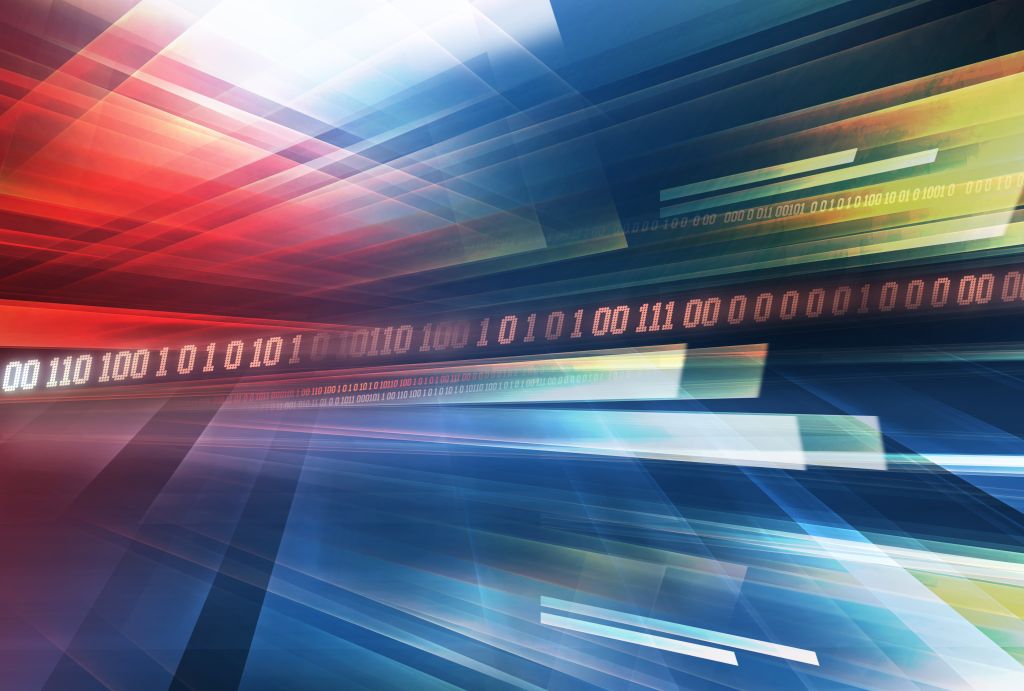
Data Preparation and Infrastructure Readiness
Data acts as the instruction set for AI models, with training datasets being crucial for model accuracy and performance. It is essential to determine where data resides, whether in the cloud or on-premise systems and assess how it can be accessed efficiently to feed AI models without creating bottlenecks. To achieve this, adopting an enterprise architecture that accounts for the geographic distribution of systems and strategically “pre-staging” data can optimize efficiency, much like content distribution networks.
Data Quality and Reference Data
Further, data readiness for AI requires a focus on two critical elements—data quality and reference data. It’s important to assess if there are robust processes to ensure data quality, which includes data cleaning, validation, and standardization. This means ensuring completeness, accuracy, and consistency in the data collected. The concept of reference data implies having an unambiguous, standardized data format across departments to avoid misinterpretations and inconsistencies. This is particularly important for cross-agency collaboration, where different departments may use the same data entities in different ways.
Data Governance and Sovereign AI
There is a need for a strong data governance framework as well that not only ensures the proper handling of data across its lifecycle but also includes aspects such as data ownership, usage policies, and reference data. This framework should establish clear data management roles and responsibilities, defining who is accountable for data quality, security, and accessibility. Agencies must consider sovereign AI, which means ensuring that the AI capabilities meet all relevant policy and statutory requirements. This involves defining operational boundaries for data and understanding the rights of the AI system to access data within specific jurisdictions.
Data governance must account for regulatory standards on data privacy, security, and provenance, particularly in a global or cloud-based setting. Government data often has complex requirements, and building compliant AI solutions means that these challenges must be addressed at every stage of the AI system’s life cycle.
5. Technology & Platforms
AI Infrastructure and Integration
AI requires a solid foundation of technology and platforms, encompassing hardware and software that underpin AI systems. Agencies must evaluate and possibly upgrade existing IT infrastructure to support new AI capabilities, including the use of APIs to connect AI tools to legacy databases or integrating AI models in stages to ensure compatibility.
Scalability and Modernization
To ensure the continued efficacy of AI systems after full deployment, agencies must implement comprehensive monitoring and evaluation frameworks. This involves tracking system performance, data quality, compliance with ethical standards, and assessing AI outcomes against key performance indicators (KPIs) relevant to the agency’s mission. Leveraging MLOps (Machine Learning Operations) tools allows for automation in deployment, monitoring, and model retraining, ensuring optimal performance.
Continuous monitoring, combined with feedback loops from end-users and system metrics, is essential for identifying issues like model drift and degraded performance. Regular updates and model retraining with new data help maintain responsiveness to evolving requirements, ensuring AI systems continue to provide valuable insights and outcomes.
6. Ethics
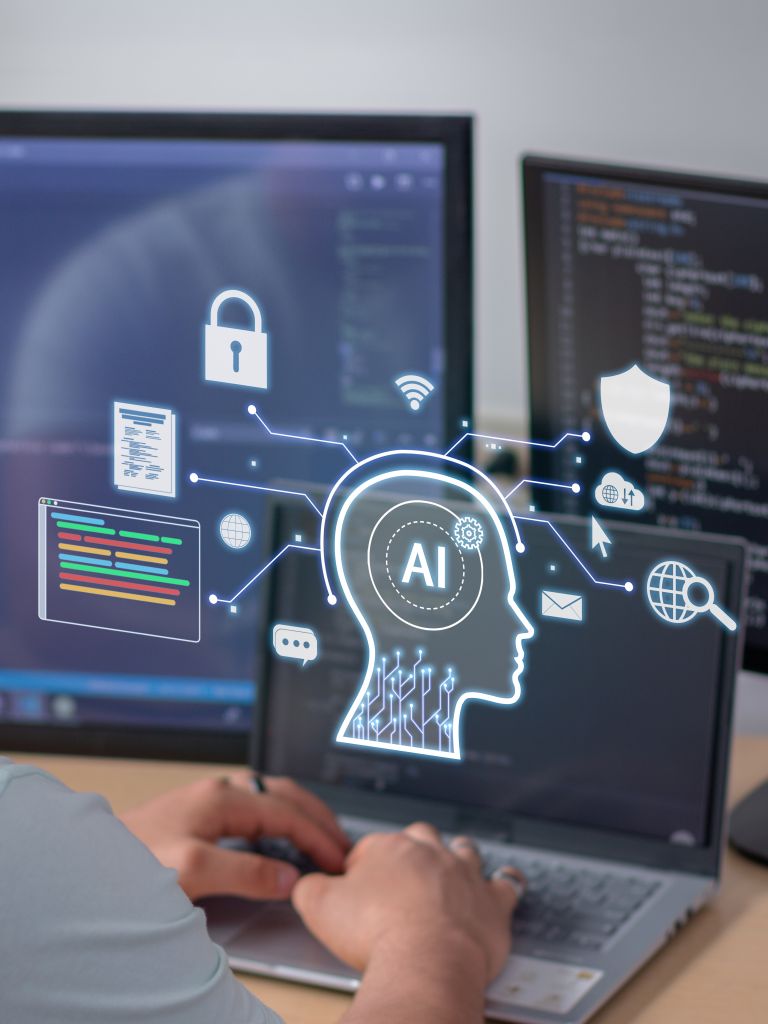
Responsible AI Implementation and Ethical Guidelines
Ethics is a critical aspect of AI readiness, particularly in government applications. Agencies must address the moral implications of AI deployment by ensuring transparency, fairness, and accountability. This involves developing frameworks to mitigate biases and protect privacy, as well as applying fairness-aware machine learning techniques.
Principles of Transparency and Accountability
To maintain public trust, government agencies must implement explainable AI (XAI) methods that make AI decisions understandable for both decision-makers and the public. Logs and audit trails should be created to enable retrospective examination of AI decisions, particularly in high-stakes domains like law enforcement and social services.
Transparency in AI processes is key to fostering trust among government employees and the public. Making AI mechanisms understandable and providing communication channels to explain outputs are crucial for building confidence in AI-driven functions, such as fraud detection and automated decision-making.
Addressing Bias and Ensuring Fairness
Bias in AI systems can lead to unfair treatment of certain groups. Agencies must use bias detection techniques, such as fairness-aware machine learning, to identify and mitigate biases in datasets and models. Applying fairness metrics during training helps prevent AI from reinforcing existing inequalities.
Ensuring fairness requires collecting diverse datasets to avoid historical biases. Testing AI models against synthetic datasets can help highlight biases and verify that AI behavior remains fair across different demographic groups.
Implementing Ethical Guidelines in AI Projects
Agencies need AI-specific ethical guidelines tailored to their domain. These should cover aspects such as informed consent for data use, ethical boundaries for AI applications, and the need for human oversight. Collaborating with stakeholders—policy experts, ethicists, and affected community members—ensures these guidelines align with public values.
Ethical oversight must be ongoing. Agencies should establish review boards that regularly assess AI deployments to ensure adherence to ethical standards throughout the project lifecycle. This includes evaluating implications as AI scales and ensuring compliance with ethical and legal frameworks.
Selecting tailored AI Solutions for diverse government needs
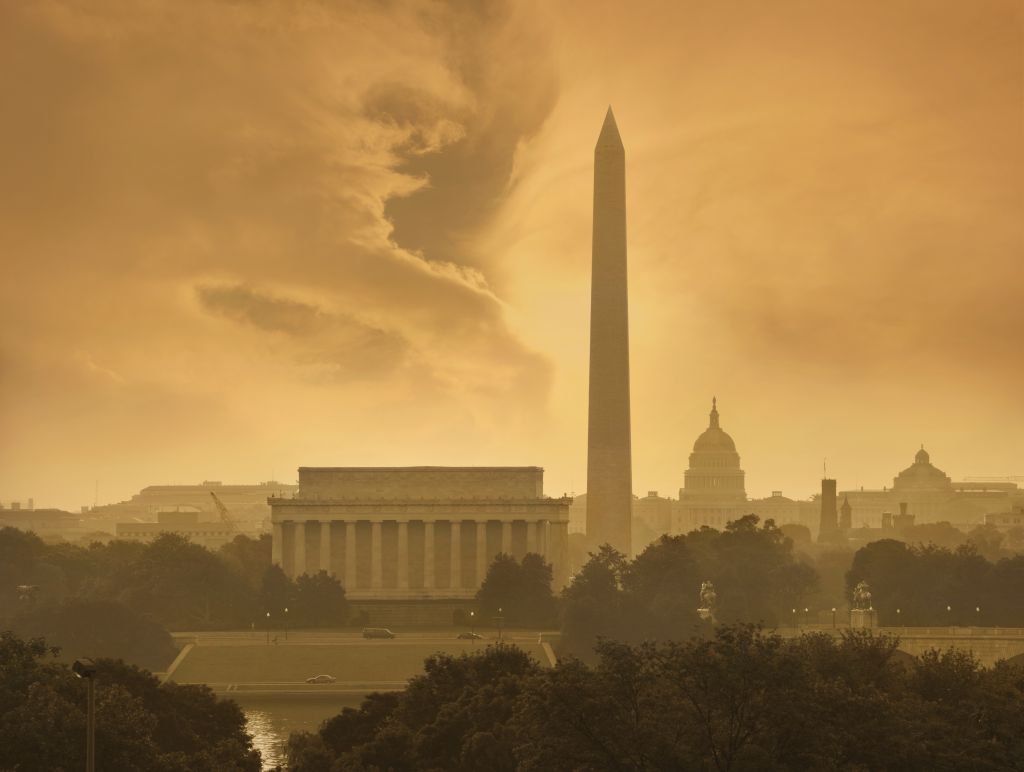
Throughout the process of assessing AI readiness, it is important to realize that different government levels, such as federal, state, and local agencies, have unique needs when implementing AI solutions.
Federal agencies often handle larger datasets and require AI systems that can operate at scale across various departments and locations. For instance, AI at the federal level may focus on enhancing national security, optimizing health services, or managing federal databases.
In contrast, state and local governments have more localized challenges, such as traffic management, resource allocation, and community services. To address these different needs, AI solutions must be tailored accordingly—focusing on scale and robustness for federal use while emphasizing adaptability and cost-effectiveness for local applications.
Customization of AI tools is crucial to address the specific requirements of different government levels. For instance, federal agencies may need AI tools integrated into nationwide systems, while state agencies may prioritize AI solutions that can adapt to local governance needs and constraints. The ability to customize AI solutions ensures that each level of government gets maximum value from AI technology, effectively aligning AI implementations with their respective missions and objectives.
A nuanced approach to AI customization helps ensure that AI initiatives are relevant, sustainable, and capable of providing tangible benefits to the public sector. For government agencies and other bodies, these tailored, flexible, and adaptable AI solutions will be key to effectively leveraging AI for service delivery and mission fulfillment.
Conclusion
Evaluating AI readiness is essential for agencies to implement AI solutions that are aligned, effective, and responsible. A comprehensive readiness assessment across areas such as strategy, infrastructure, data, and ethics provides a foundation for successful government AI integration that enhances mission delivery.
To advance AI adoption, agencies should prioritize aligning AI projects with strategic goals, modernizing outdated systems, ensuring data governance, and addressing ethical considerations. Tailored solutions are crucial for addressing the unique needs of federal, state, local, and other public sector organizations.
TechSur assists agencies at every stage of their AI readiness journey—from developing tailored strategies and solutions to executing pilot projects—helping you achieve your goals with effective and sustainable AI integration.
References: AI Operating Model from Deloitte