Google’s Gemini Ultra incurred an estimated $191 million in compute expenses during its training phase, highlighting the substantial resources required for developing frontier-scale large language models. In contrast, small language models (SLMs) with 100 to 500 million parameters typically require significantly fewer resources, with estimated training costs ranging between $50,000 and $250,000. These contrasting figures demonstrate the vast disparity in training costs between large-scale and small-scale language models.
Reevaluating Model Size: Why Larger Isn’t Always the Best Path
When evaluating AI adoption, federal agencies must prioritize mission alignment over model scale. Unlike commercial tech companies pursuing general-purpose AI, government projects often have highly specific scopes, such as form processing, case triage, or domain-specific analytics, that do not require the vast generalization capabilities of massive language models. As highlighted during the ATARC Public Sector AI Summit, agency leaders emphasized the importance of asking fundamental questions upfront:
“Understand what model we need and why; do we need to make our own model; do we need to train the model, or can we use a pre-trained small language model?”
In tightly regulated environments with constraints on data privacy, security, and explainability, deploying a right-sized, domain-focused model is not only more efficient but often more compliant.
Beyond operational scope, computational and financial overhead must also guide model selection. While larger models can excel in broad, open-ended tasks, they demand significant infrastructure, specialized hardware, and ongoing tuning costs that quickly escalate and complicate secure deployments. Smaller, specialized SLMs dramatically reduce training timelines, simplify deployment within FedRAMP or FISMA frameworks, and allow teams to focus on achieving targeted performance benchmarks rather than managing unnecessary complexity. It is precisely these smaller models that offer federal agencies a strategic advantage: the ability to balance accuracy, speed, cost, and compliance by tailoring AI solutions directly to the mission need.
Utilizing Pre-Trained AI for Swift, Compliant Deployments
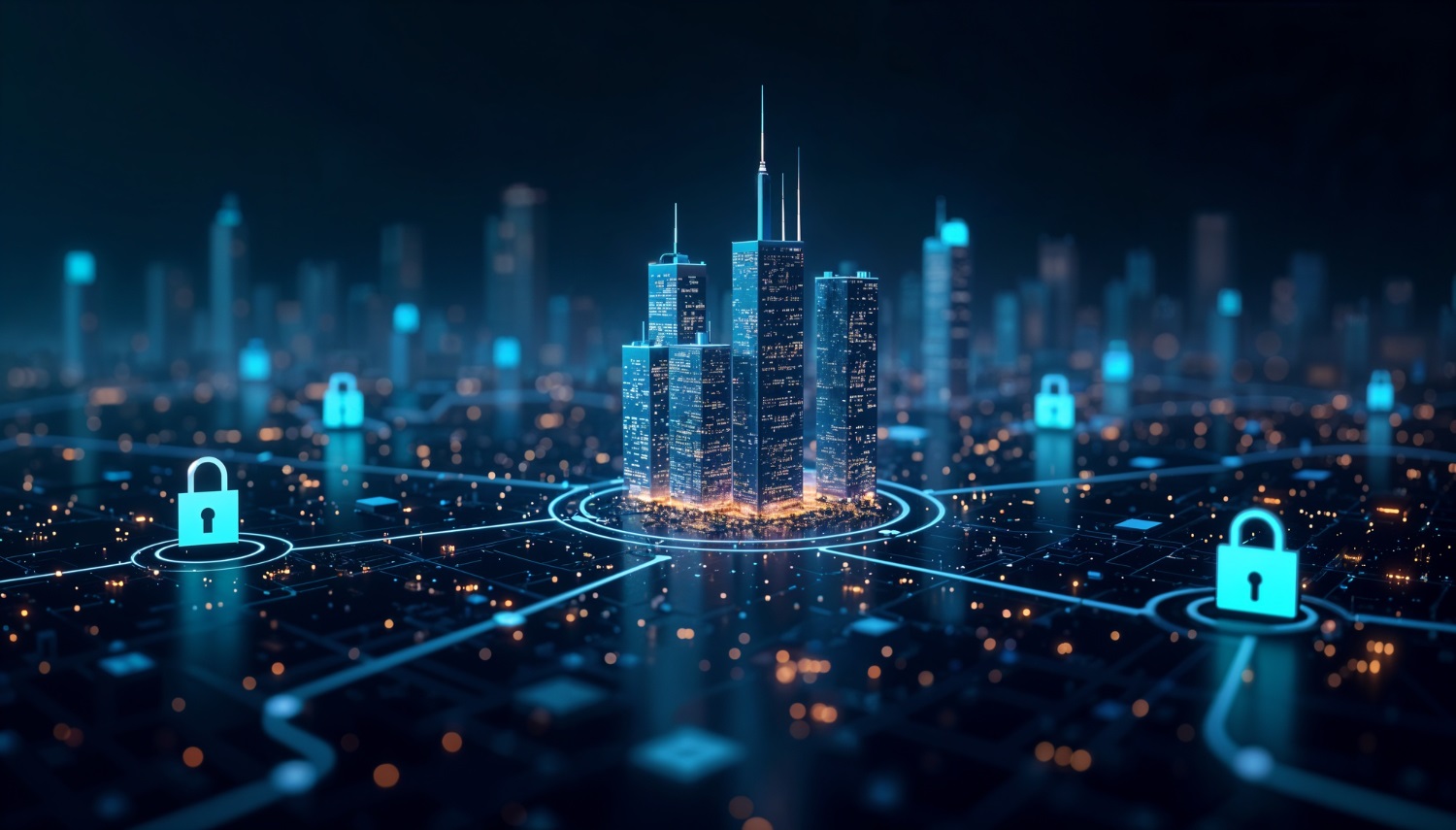
Pre-trained SLMs can offer federal agencies a fast, efficient path to AI adoption. Ready-made models for tasks like summarization, classification, and entity recognition allow teams to shorten deployment timelines and reduce the complexity of custom model development. Minimal fine-tuning is often sufficient, enabling agencies to tailor models to specific missions with lower costs and faster results. This approach is aligned with the NIST AI Risk Management Framework (AI RMF 1.0), which encourages the use of modular, adaptable AI systems to lower technical complexity and enable faster, more mission-appropriate deployments.
SLMs also support stronger security and compliance postures. Their smaller infrastructure demands limit data movement and reduce exposure risks, making integration into federal environments more straightforward. By combining lightweight models with Retrieval-Augmented Generation (RAG) techniques, agencies can further enhance performance by dynamically retrieving domain-specific context, achieving high accuracy without the resource burden of managing large-scale models.
Capturing the Untapped Advantages of Smaller Language Models
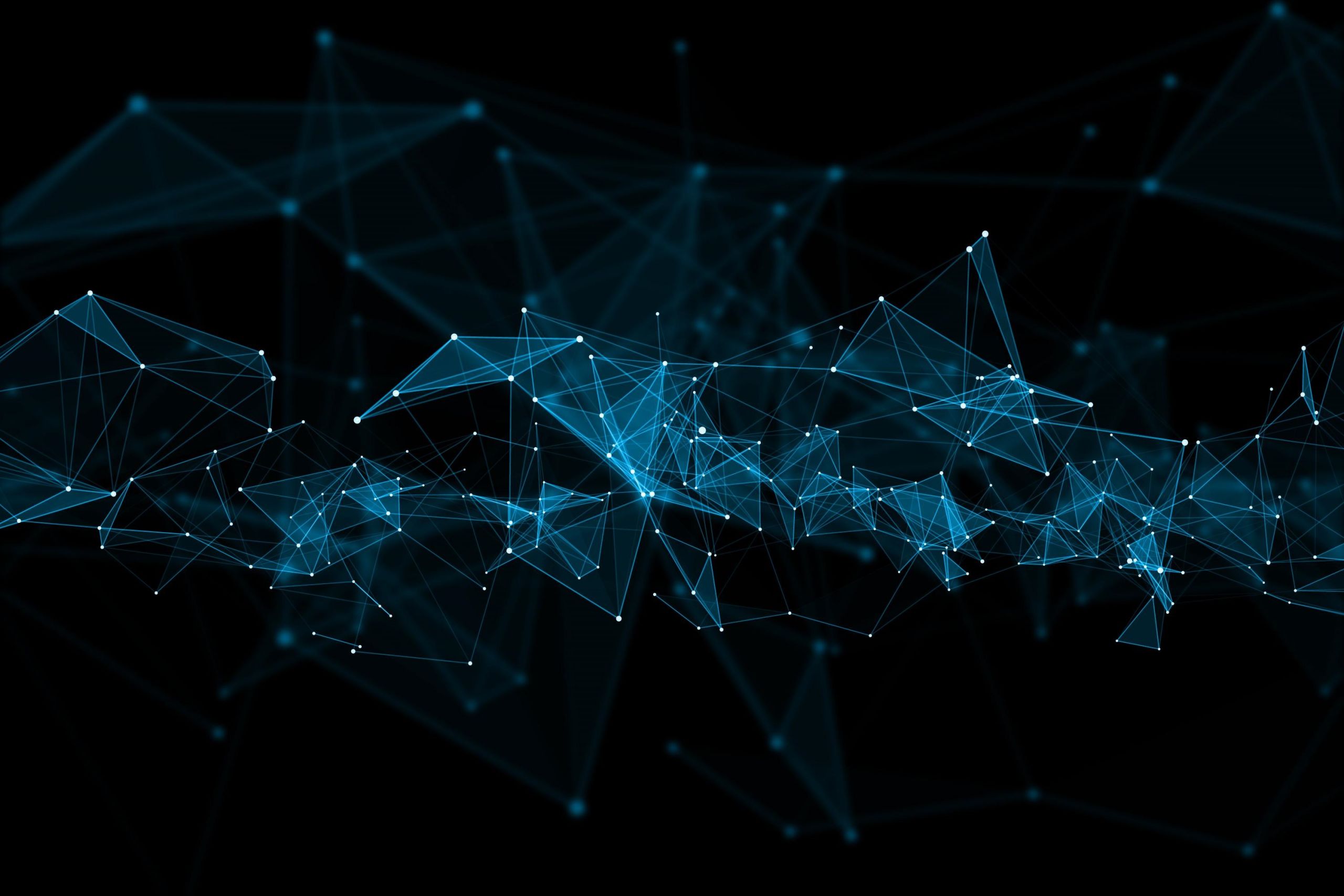
Beyond faster deployment and compliance benefits, smaller language models offer federal agencies significant advantages in resource efficiency and adaptability. Due to their reduced computational footprint, SLMs can operate on smaller infrastructure setups, lowering overall infrastructure costs and energy consumption. This efficiency makes them particularly well-suited for deployment on edge devices, remote installations, or within highly constrained environments where full-scale cloud or data center access may be limited.
Small language models also enable rapid iteration and versioning, allowing agencies to update, fine-tune, and re-deploy AI solutions as regulatory requirements, mission needs, or operational contexts evolve. This agility is critical in public-sector environments where policies and compliance frameworks frequently undergo revision. For example, the U.S. Department of Veterans Affairs (VA) has been exploring lightweight, fine-tuned AI models to assist with clinical note summarization and patient triage, allowing faster deployment cycles and easier retraining in response to evolving healthcare protocols.
From a governance and oversight perspective, smaller models offer enhanced control and transparency. Their simpler architectures make it easier to interpret, audit, and validate outputs—an essential capability in government sectors where explainability and accountability are mandated by law and public trust. Furthermore, by focusing on narrower, mission-specific tasks, SLMs can often deliver higher accuracy rates compared to generalized large models, particularly in use cases such as form processing, case triaging, and domain-specific knowledge retrieval.
Conclusion
Smaller Language Models (SLMs) can offer a balanced approach to public sector AI initiatives. They deliver mission-focused performance, reduced costs, faster deployments, and strong compliance support compared to larger, more complex models. By considering the scope of the mission, security constraints, and resource availability, government agencies can harness the benefits of both small and large models without overextending.
TechSur Solutions stands ready to guide agencies through this journey, ensuring that every AI implementation is both technologically robust and strategically aligned with public-sector objectives. We take advantage of the advanced capabilities available through hyperscaler platforms while also delivering domain-tuned SLMs that are right-sized for specific missions. This hybrid approach ensures agencies can access scalable AI innovations while maintaining the control, efficiency, and compliance required in government environments.